本篇我们继续聊聊kombu这个python实现的消息库中的一些高级算法实现。
Celery是一款非常简单、灵活、可靠的分布式系统,可用于处理大量消息,并且提供了一整套操作此系统的工具。Celery 也是一款消息队列工具,可用于处理实时数据以及任务调度。
本文是是celery源码解析的第四篇,在前3篇里分别介绍了vine, py-amqp和kombu:
- 神器 celery 源码解析- vine实现Promise功能
- 神器 celery 源码解析- py-amqp实现AMQP协议
- 神器 celery 源码解析- kombu,一个python实现的消息库
kombu中的高级算法和各种排序算法不一样,都是解决一些具体的业务问题,非常有用。本文包括下面几个部分:
- LRU缓存淘汰算法
- 令牌桶限流算法
- Round-Robin调度算法
- LamportClock时间戳算法
- LaxBoundedSemaphore有限信号量算法
LRU缓存淘汰算法
缓存,顾名思义,就是将计算结果暂时存起来,以供后期使用,这样可以省去重复计算的工作。比如我们计算斐波那契数列的递归算法:
1
2
3
4
5
|
# 根据定义递归求解
def fib(n):
if n <= 1:
return n
return fib(n - 1) + fib(n - 2)
|
我们求n为5的数,展开数学公式大概如下(这里简化python函数fib名称为数学函数f):
1
2
3
4
5
|
f(5)=f(4) +f(3)
=f(3) +f(2) +f(2) +f(1)
=f(2) +f(1)+f(1)+f(0)+f(1)+f(0)+f(1)
=f(1)+f(0)+f(1)+f(1)+f(0)+f(1)+f(0)+f(1)
=5
|
根据数学公式,我们可以知道,在执行f(5)过程中,重复执行了5次f(1), 3次f(0)。要提高执行效率,就可以用到缓存。最简单的实现版本:
1
2
3
4
5
6
7
8
9
10
11
|
# 根据定义递归求解
cache = {}
def fib_v1(n):
if n in cache:
return cache[n]
if n <= 1:
result = n
else:
result = fib(n - 1) + fib(n - 2)
cache[n] = result
return result
|
这种实现方式有2个弊端,一个是依赖一个外部的cache变量,另一个是cache功能和fib函数绑定,还需要修改fib函数。我们可以通过一个装饰器实现这个cache,而不用改动fib函数:
1
2
3
4
5
6
7
8
9
10
11
12
|
def cache_decorator(fun):
_cache = {}
def wrapper(*args, **kwargs):
if args in _cache:
return _cache[args]
else:
ret = fun(*args, **kwargs)
_cache[args] = ret
return ret
return wrapper
|
使用的时候可以直接给fib函数添加上装饰器:
1
2
3
|
@cache_decorator
def fib(n):
...
|
这种缓存实现实现方式,还是会有问题:无法进行清理,内存会持续增长。编程中有一句话是: 命名和缓存失效是计算机科学里面最难应对的两件事。关于缓存淘汰有各种算法,请见参考链接,我这里重点介绍一下LRU和LFU。
- LRU(Least recently used)最早使用淘汰算法,核心特点是: 最早的数先淘汰
- LFU(Least-frequently used)最少使用淘汰算法, 核心特点是: 最少的数先淘汰
关于LRU,在我之前介绍tinydb时候有过介绍。其中的实现如下:
1
2
3
4
5
6
7
8
9
10
11
12
13
14
15
16
17
18
19
20
21
|
class LRUCache(abc.MutableMapping, Generic[K, V]):
def __init__(self, capacity=None):
self.capacity = capacity # 缓存容量
self.cache = OrderedDict() # 有序字典
def get(self, key: K, default: D = None) -> Optional[Union[V, D]]:
value = self.cache.get(key) # 从换成获取
if value is not None:
del self.cache[key]
self.cache[key] = value # 更新缓存顺序
return value
return default
def set(self, key: K, value: V):
if self.cache.get(key):
del self.cache[key]
self.cache[key] = value # 更新缓存顺序及值
else:
self.cache[key] = value
if self.capacity is not None and self.length > self.capacity:
self.cache.popitem(last=False) # 淘汰最古老的数据
|
LRU的特点只要保持缓存数据是有序的, 我们甚至不需要自己实现,使用系统functools中的实现:
1
2
3
4
5
|
from functools import lru_cache
@lru_cache()
def fib(n):
...
|
kombu中给我们提供了一个线程安全的版本, 主要实现:
1
2
3
4
5
6
7
8
9
10
11
12
13
14
15
16
17
18
19
20
21
22
|
# kombu-5.0.0/kombu/utils/functional.py
class LRUCache(UserDict):
"""LRU Cache implementation using a doubly linked list to track access.
"""
def __init__(self, limit=None):
self.limit = limit
self.mutex = threading.RLock()
self.data = OrderedDict()
def __getitem__(self, key):
with self.mutex:
value = self[key] = self.data.pop(key)
return value
def __setitem__(self, key, value):
# remove least recently used key.
with self.mutex:
if self.limit and len(self.data) >= self.limit:
self.data.pop(next(iter(self.data)))
self.data[key] = value
...
|
上面代码在设置和获取数据时候都先获取锁,然后再进行数据操作。
关于缓存使用,除了通过业务场景判断适用那种淘汰算法外,还可以使用具体的缓存命中率指标进行分析:
1
2
3
4
5
6
7
8
9
10
11
12
13
14
15
16
17
18
19
20
21
22
23
24
25
26
27
28
29
30
31
32
33
34
35
36
37
38
|
def memoize(maxsize=None, keyfun=None, Cache=LRUCache):
"""Decorator to cache function return value."""
def _memoize(fun):
mutex = threading.Lock()
cache = Cache(limit=maxsize)
@wraps(fun)
def _M(*args, **kwargs):
if keyfun:
key = keyfun(args, kwargs)
else:
key = args + (KEYWORD_MARK,) + tuple(sorted(kwargs.items()))
try:
with mutex:
value = cache[key]
except KeyError:
value = fun(*args, **kwargs)
# 未命中需要执行函数
_M.misses += 1
with mutex:
cache[key] = value
else:
# 命中率增加
_M.hits += 1
return value
def clear():
"""Clear the cache and reset cache statistics."""
# 清理缓存及统计
cache.clear()
_M.hits = _M.misses = 0
# 统计信息
_M.hits = _M.misses = 0
_M.clear = clear
_M.original_func = fun
return _M
return _memoize
|
memoize的实现并不复杂,增加了hits/misses数据,可以统计分析缓存的命中率,帮助正确使用LRU缓存。还添加了clear接口,可以在需要的时候对缓存直接进行清理。
注意memoize使用了一个锁,在LRUCache还是使用了一个锁,这个锁的使用,我们以后再讲。
令牌桶限流算法
限流是指在系统面临高并发、大流量请求的情况下,限制新的流量对系统的访问,从而保证系统服务的安全性。常用的限流算法有计数器、漏斗算法和令牌桶算法。其中计数器算法又分固定窗口算法、滑动窗口算法,后者我们在TCP协议中经常会碰到。
算法中存在一个令牌桶,以恒定的速率向令牌桶中放入令牌。当请求来时,会首先到令牌桶中去拿令牌,如果拿到了令牌,则该请求会被处理,并消耗掉令牌;如果拿不到令牌,则该请求会被丢弃。当然令牌桶也有一定的容量,如果满了令牌就无法放进去了,这样算法就有限流作用。又因为令牌产生的速率是很定的,如果消费速率较低,桶里会额外缓存一部分令牌,用于应对流量突发时候的消耗。下面是算法的示意图:
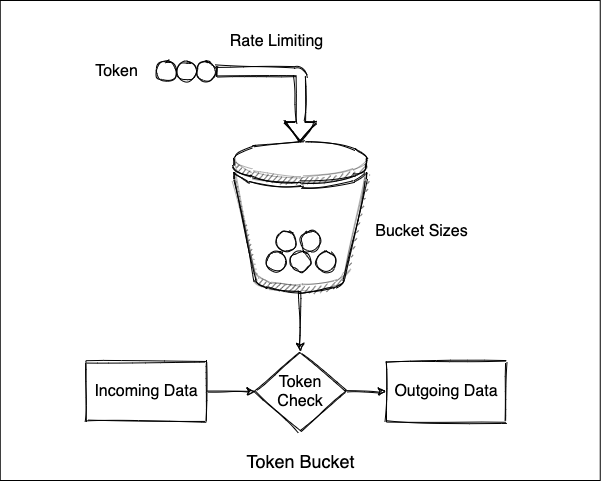
我们具体看看kombu中提供的实现。TokenBucket类:
1
2
3
4
5
6
7
8
9
10
11
12
13
14
15
16
17
18
19
20
21
22
23
24
25
26
27
|
class TokenBucket:
#: The rate in tokens/second that the bucket will be refilled.
fill_rate = None
#: Maximum number of tokens in the bucket.
capacity = 1
#: Timestamp of the last time a token was taken out of the bucket.
timestamp = None
def __init__(self, fill_rate, capacity=1):
# 容量上限
self.capacity = float(capacity)
# 剩余令牌数,初始等于容量上限
self._tokens = capacity
# 填充率
self.fill_rate = float(fill_rate)
self.timestamp = monotonic()
# 数据容器
self.contents = deque()
def add(self, item):
self.contents.append(item)
def pop(self):
# 先进先出
return self.contents.popleft()
|
代码包括:
- 令牌速率fill_rate
- 桶的容量上限
- 一个时间戳
- 剩余令牌数
- 算法提供了一个基于双端队列的数据容器,可以对容器进行先进先出操作
令牌桶是否可用的判断:
1
2
3
4
5
6
7
8
9
10
11
12
13
14
15
16
17
18
19
20
21
22
23
24
25
26
27
|
def can_consume(self, tokens=1):
"""Check if one or more tokens can be consumed.
Returns:
bool: true if the number of tokens can be consumed
from the bucket. If they can be consumed, a call will also
consume the requested number of tokens from the bucket.
Calls will only consume `tokens` (the number requested)
or zero tokens -- it will never consume a partial number
of tokens.
"""
if tokens <= self._get_tokens():
# 消费n个令牌
self._tokens -= tokens
return True
return False
def _get_tokens(self):
if self._tokens < self.capacity:
# 记录当前时间
now = monotonic()
# 计算已经流失的令牌数量
delta = self.fill_rate * (now - self.timestamp)
# 更新容量上限或者剩余令牌和流失数量之和
self._tokens = min(self.capacity, self._tokens + delta)
self.timestamp = now
return self._tokens
|
我们可以看到,算法在进行令牌消费判断的同时,还会对桶的剩余流量进行自校正,很巧妙。
TokenBucket的使用在ConsumerMixin的run方法中。创建了一个速率为1的令牌桶,然后持续的进行消费。如果有令牌则消费消费者上的消息;如果没有令牌则进行休眠
1
2
3
4
5
6
7
8
9
10
11
12
13
14
15
16
17
18
19
|
#ch23-celery/kombu-5.0.0/kombu/mixins.py:240
class ConsumerMixin:
def run(self, _tokens=1, **kwargs):
restart_limit = TokenBucket(1)
...
# 无限循环
while not self.should_stop:
try:
# 有令牌消费
if restart_limit.can_consume(_tokens): # pragma: no cover
for _ in self.consume(limit=None, **kwargs):
pass
else:
# 没浪费休眠
sleep(restart_limit.expected_time(_tokens))
except errors:
...
|
其中的休眠时间,是由令牌桶根据期望值计算得来:
1
2
3
4
5
6
7
8
9
|
def expected_time(self, tokens=1):
"""Return estimated time of token availability.
Returns:
float: the time in seconds.
"""
_tokens = self._get_tokens()
tokens = max(tokens, _tokens)
return (tokens - _tokens) / self.fill_rate
|
Round-Robin调度算法
Round-Robin调度算法,最常见的大概是在nginx。Round-Robin方式可让nginx将请求按顺序轮流地分配到后端服务器上,它均衡地对待后端的每一台服务器,而不关心服务器实际的连接数和当前的系统负载,循环往复。在kombu中也提供了几种类似的调度算法:
我们先看Round-Robin方式:
1
2
3
4
5
6
7
8
9
10
11
12
13
14
15
16
17
18
19
20
21
22
23
24
25
26
|
class round_robin_cycle:
"""Iterator that cycles between items in round-robin."""
"""轮询调度算法"""
def __init__(self, it=None):
self.items = it if it is not None else []
def update(self, it):
"""Update items from iterable."""
"""更新列表"""
self.items[:] = it
def consume(self, n):
"""Consume n items."""
"""消费n个元素"""
return self.items[:n]
def rotate(self, last_used):
"""Move most recently used item to end of list."""
"""旋转:把最后一个元素放到列表某尾"""
items = self.items
try:
items.append(items.pop(items.index(last_used)))
except ValueError:
pass
return last_used
|
算法实现很简单,就是一个有序队列,可以每次消费前n个有序元素,并且可以将最近使用的元素旋转到队尾。下面是旋转的单元测试:
1
2
3
4
5
6
7
8
9
10
11
|
def test_round_robin_cycle():
it = cycle_by_name('round_robin')(['A', 'B', 'C'])
assert it.consume(3) == ['A', 'B', 'C']
it.rotate('B')
assert it.consume(3) == ['A', 'C', 'B']
it.rotate('A')
assert it.consume(3) == ['C', 'B', 'A']
it.rotate('A')
assert it.consume(3) == ['C', 'B', 'A']
it.rotate('C')
assert it.consume(3) == ['B', 'A', 'C']
|
还有一种公平循环的调度算法:
1
2
3
4
5
6
7
8
9
10
11
12
13
14
15
16
17
18
|
class FairCycle:
"""Cycle between resources.
Consume from a set of resources, where each resource gets
an equal chance to be consumed from.
Arguments:
fun (Callable): Callback to call.
resources (Sequence[Any]): List of resources.
predicate (type): Exception predicate.
"""
def __init__(self, fun, resources, predicate=Exception):
self.fun = fun
self.resources = resources
self.predicate = predicate
# 初始位置
self.pos = 0
|
FairCycle是一种资源之间公平循环的调度算法, 构造函数中:
使用的方式是使用get方法传入回调:
1
2
3
4
5
6
7
8
9
10
11
12
13
14
15
16
17
18
19
20
21
22
23
24
25
26
27
|
def _next(self):
while 1:
try:
resource = self.resources[self.pos]
# 位置加1
self.pos += 1
return resource
except IndexError:
# 到尾部后,重置位置
self.pos = 0
if not self.resources:
raise self.predicate()
def get(self, callback, **kwargs):
"""Get from next resource."""
# 无限重试
for tried in count(0): # for infinity
# 获取资源
resource = self._next()
try:
# 利用资源
return self.fun(resource, callback, **kwargs)
except self.predicate:
# reraise when retries exchausted.
# 容错上限
if tried >= len(self.resources) - 1:
raise
|
调度主要体现再获取资源的next函数上,没次获取资源后位置标志进行后移,到尾部后在重置到0,继续下一轮循环。算法还可以对资源进行容错,也就是如果获取到的资源无法正常使用,还可以尝试使用下一个资源进行重试。
LamportClock算法
兰波特时间戳算法(LamportClock),使用逻辑时间戳作为值的版本以允许跨服务器对值进行排序,是解决分布式系统时间一致的重要算法。
服务器上的系统时间,使用物理的晶体振荡测量,会有不准的情况。我们会经常遇到服务器或者快或者慢的情况,一般使用NTP服务,来和互联网上的某个时间源进行同步。如果本地时间提前了,进行联网校时后,会出现本地时间倒退的问题。而对于两台不同的服务器上,要进行时间统一,就更不能使用系统时间。
兰波特时间戳算法,原理如下:
- 维护一个数字来表示时间戳,并且在每个集群节点都维护一个 Lamport 时钟的实例。
- 如果事件在节点内发生,时间戳加1
- 事件要发送到远端,则在消息总带上时间戳
- 接收到远端的消息,时间戳 = Max(本地时间戳,消息中的时间戳) + 1(进行校正跳跃)
这个过程,可以看下面的图示:
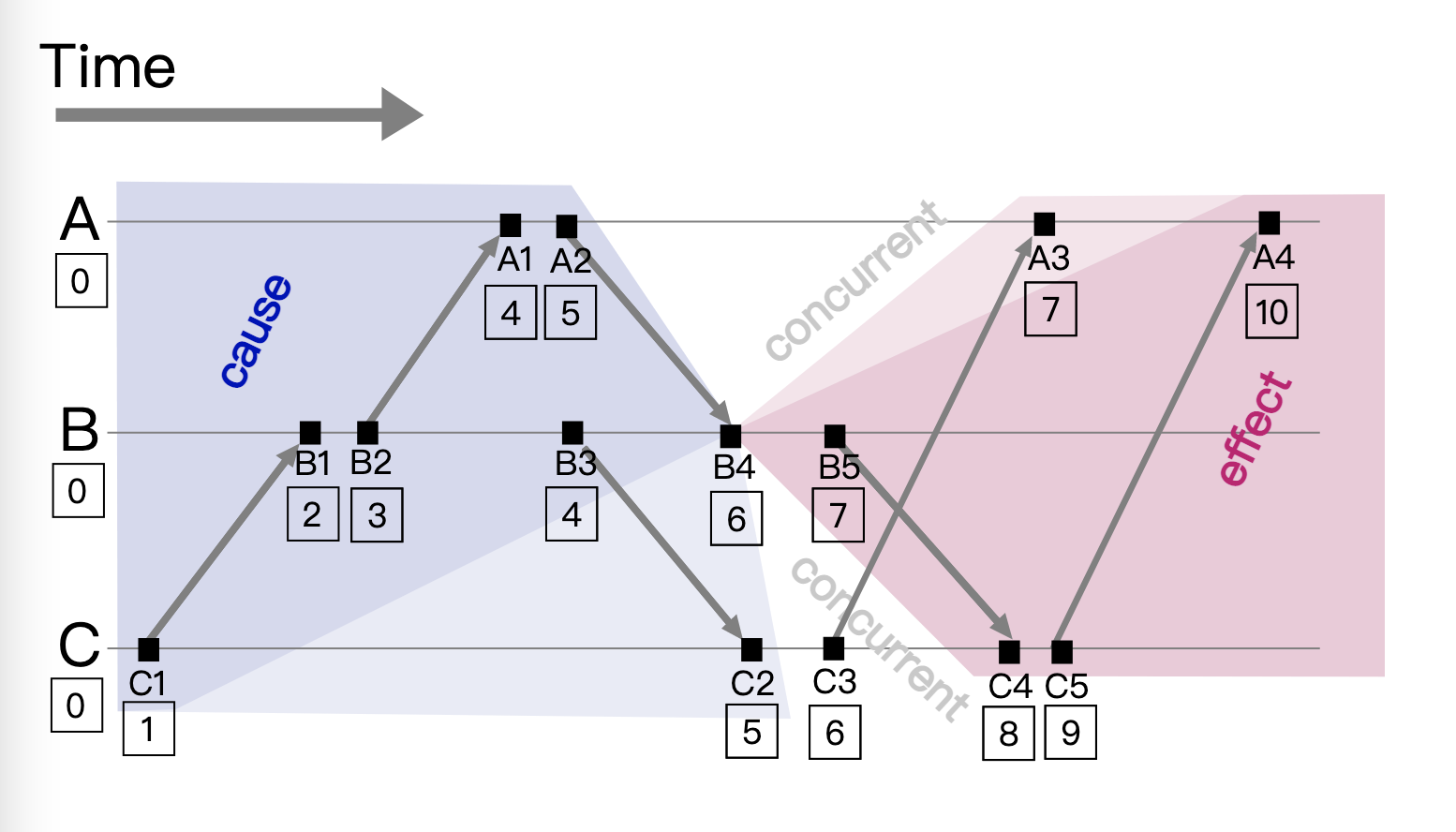
从图中可以看到下面两点:
- 对于每个节点的事件时间,都是递增有序的,比如A是[4,5,7,10], B节点是[2,3,4,6,7], C节点是[1,5,6,8,9]
- 时间戳不是全局唯一,不同节点之间会存在序号重复,比如4号消息在A和B节点都存在,5号消息在A和C节点存在
了解算法的场景和原理后,我们再来看算法的实现。
1
2
3
4
5
6
7
8
9
10
11
12
13
14
15
16
17
|
class LamportClock:
#: The clocks current value.
value = 0
def __init__(self, initial_value=0, Lock=Lock):
self.value = initial_value
self.mutex = Lock()
def adjust(self, other):
with self.mutex:
value = self.value = max(self.value, other) + 1
return value
def forward(self):
with self.mutex:
self.value += 1
return self.value
|
算法的实现其实非常简单,就是转发的时候时间戳+1;收到消息后进行校正,这个过程中使用线程锁,保证本地的有序。
LaxBoundedSemaphore有限信号量算法
前面讲的几种算法,都是基于线程锁实现。使用锁会降低效率,如果在协程中,可以使用无锁的方案,会更高效。kombu的LaxBoundedSemaphore实现,可以作为一种参考。
我们先看使用示例:
1
2
3
4
5
6
7
8
9
10
11
12
13
14
15
16
17
|
>>> from future import print_statement as printf
# ^ ignore: just fooling stupid pyflakes
>>> x = LaxBoundedSemaphore(2)
>>> x.acquire(printf, 'HELLO 1')
HELLO 1
>>> x.acquire(printf, 'HELLO 2')
HELLO 2
>>> x.acquire(printf, 'HELLO 3')
>>> x._waiters # private, do not access directly
[print, ('HELLO 3',)]
>>> x.release()
HELLO 3
|
示例展示了几步:
- 创建一个大小为2的LaxBoundedSemaphore信号量
- 申请信号,并且执行print函数,可以立即执行
- 继续申请信号执行print函数,也可以立即执行
- 再申请信号执行print函数,这时候由于信号超标,函数不会立即执行
- 手工释放信号量,最后一次申请的print函数自动执行
下面是具体的实现,LaxBoundedSemaphore的构造函数:
1
2
3
4
5
6
7
8
|
class LaxBoundedSemaphore:
def __init__(self, value):
# 信号容量
self.initial_value = self.value = value
# 使用双端队列,FIFO
self._waiting = deque()
self._add_waiter = self._waiting.append
self._pop_waiter = self._waiting.popleft
|
申请执行回调函数,会进行信号判断,信号充足会执行行回调并消减一次信号量;信号量不足则将函数及参数放入代办的队列:
1
2
3
4
5
6
7
8
9
10
11
12
13
14
15
16
17
18
19
20
21
22
|
def acquire(self, callback, *partial_args, **partial_kwargs):
"""Acquire semaphore.
This will immediately apply ``callback`` if
the resource is available, otherwise the callback is suspended
until the semaphore is released.
Arguments:
callback (Callable): The callback to apply.
*partial_args (Any): partial arguments to callback.
"""
value = self.value
if value <= 0:
# 容量不够的时候先暂存执行函数,并不更改可用数量
self._add_waiter((callback, partial_args, partial_kwargs))
return False
else:
# 可用数量-1
self.value = max(value - 1, 0)
# 直接执行函数
callback(*partial_args, **partial_kwargs)
return True
|
使用release时候会取出头部的代办函数,并进行执行,此时信号量不增不减。如果代办全部执行完成后,则逐步恢复信号量到默认值:
1
2
3
4
5
6
7
8
9
10
11
12
13
14
15
|
def release(self):
"""Release semaphore.
Note:
If there are any waiters this will apply the first waiter
that is waiting for the resource (FIFO order).
"""
try:
waiter, args, kwargs = self._pop_waiter()
except IndexError:
# 无缓存则只增加可用数量
self.value = min(self.value + 1, self.initial_value)
else:
# 有缓存则执行第一个缓存,可用数量不变还是小于0
waiter(*args, **kwargs)
|
小结
本篇文章,我们学习了5种实用的业务算法。LRU缓存淘汰算法,可以对缓存中最早的数据进行淘汰。令牌桶限流算法,可以协助进行服务流量限流,较好的保护后端服务,避免突发流量的到时的崩溃。Round-Robin调度算法,可以进行负载的均衡,保障资源的平衡使用。LamportClock时间戳算法,可以在分布式系统中,进行不同服务之间的有序时间戳同步。LaxBoundedSemaphore有限信号量算法,是一种无锁算法,可高效的提供资源使用控制。
小技巧
kombu中提供了一个自动重试算法,可以作为重试算法的模版:
1
2
3
4
5
6
7
8
9
10
11
12
13
14
15
16
17
18
19
20
21
22
23
24
25
|
# kombu-5.0.0/kombu/utils/functional.py
def retry_over_time(fun, catch, args=None, kwargs=None, errback=None,
max_retries=None, interval_start=2, interval_step=2,
interval_max=30, callback=None, timeout=None):
kwargs = {} if not kwargs else kwargs
args = [] if not args else args
interval_range = fxrange(interval_start,
interval_max + interval_start,
interval_step, repeatlast=True)
# 超时时间
end = time() + timeout if timeout else None
for retries in count():
try:
return fun(*args, **kwargs)
except catch as exc:
# 超过次数
if max_retries is not None and retries >= max_retries:
raise
# 超过时间
if end and time() > end:
raise
...
# 休眠
sleep(1.0)
|
从模版可以看到重试时候使用次数和超时时间两个维度进行跳出(不可能无限重试):
- 使用count()进行无限循环
- 使用time()进行超时限定
- 使用max_retries容错上限次数限定
- 每次错误后,都休眠一段时间,给被调用方机会,提高下一次成功的概率。
实际上关于休眠时间,也有一些更复杂的算法,比如线性递增之类,这里使用了固定间隔的休眠
参考链接